Société de Transport de Montréal (STM)

The context
Did you know that it’s possible for companies to experiment (affordably) with state of the art technology, like Boston Dynamics’ Spot robot?
Well, the Société de transport de Montréal (STM), who is responsible for operating bus, métro and paratransit services in the city, did just that. Their iconic metro system, with stations recognized for their distinct architecture and design, launched in 1966 just in time for Expo 67. With so much technological change over the last 50 years, it comes at no surprise that continued innovation is imperative for the public transport agency as they move 680 000 people along 71 kilometers of tracks, daily.
The STM became aware of Osedea’s role as a Boston Dynamics solutions partner for Canada, and wanted to know how Spot might be used to automate some of their processes and help improve their customer experience. As a recipient of a grant called “Soutien aux projets d’innovation” from Investissement Québec, Osedea in collaboration with the STM, were to execute an experimental pilot that would assist STM station workers with sanitation.
Here's how we approached this project with Spot.
Project details
The challenge
The idea was to have Spot perform automated and autonomous inspections of Bonaventure metro station after public transit customers went home for the night. To gain sufficient visibility, each evening Spot would snap photos of the Montmorency platform every 10 meters from different angles. The goal was to get as close as possible to capturing 360 degrees of the space to find issues, such as the status of lightbulbs (i.e. if they were still lit, or burnt out), as well as the absence or presence of trash, stickers and graffiti.
We faced several unique challenges:
- Because the station is a public space with no room for infrastructure adjustments, we could not have doors automatically open for Spot, nor could Spot pass autonomously through the turnstiles (it had to be let through by an operator).
- We had to work within rigid time constraints between 1am - 4am when the station was clear, with one robot that required 90 minutes of operating time and two hours of charge time.
- In order to cover the entire station, Spot would need to capture more than 300 photos per platform, which is a large volume of data.
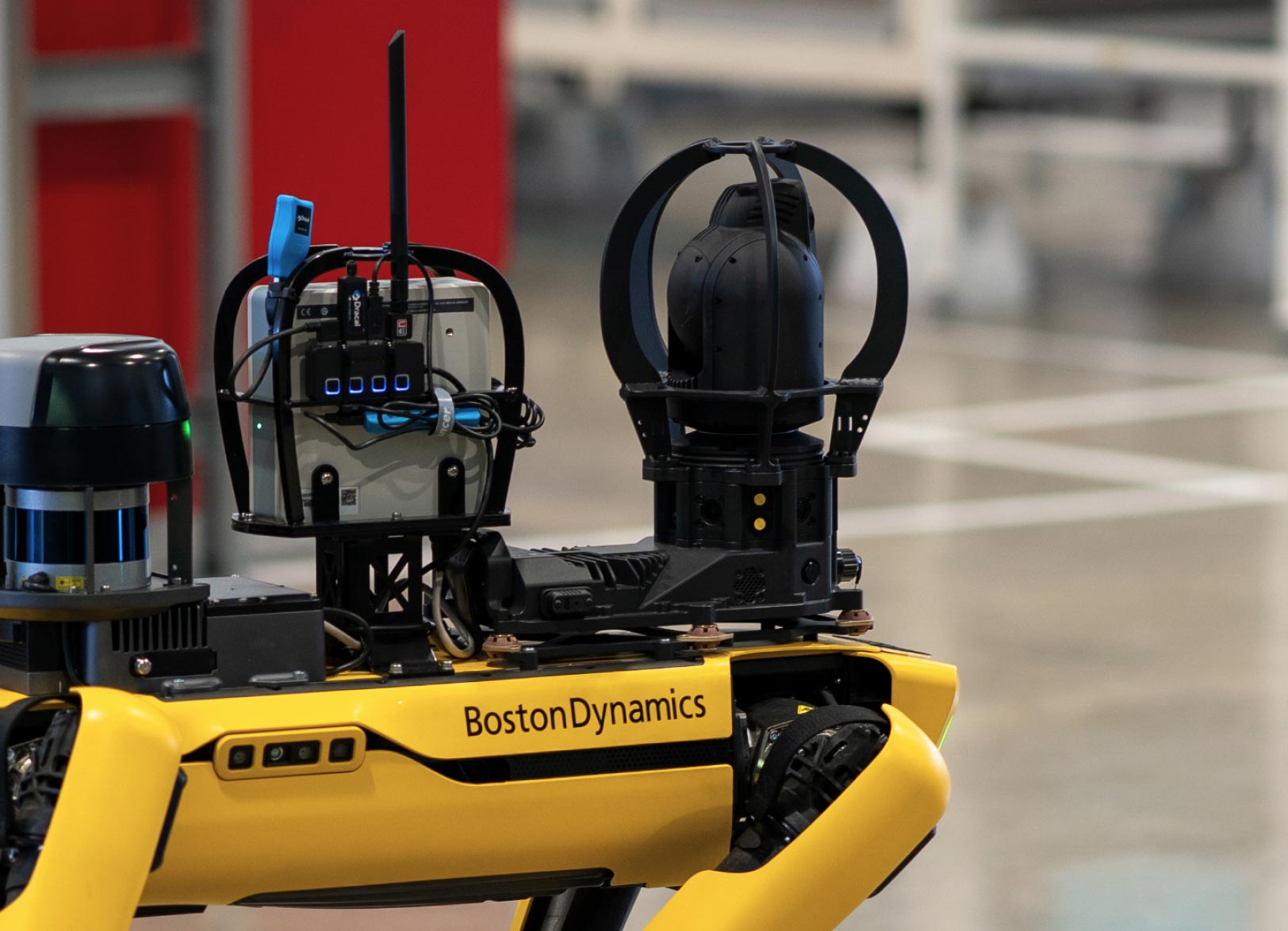
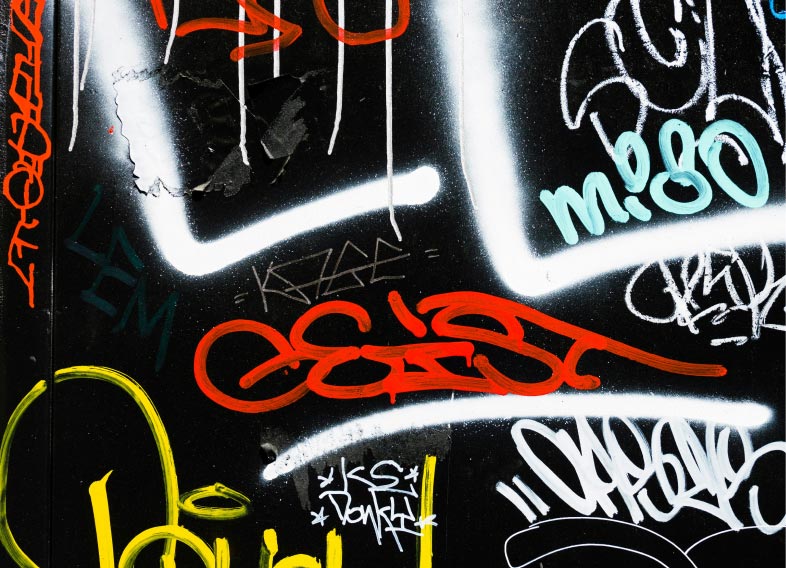
The task at hand
For equipment, we leveraged Spot Explorer (the robot), Spot CORE (an onboard computer for custom logic), and Spot CAM+IR (the camera).
While Spot may not be able to remove graffiti, change broken lightbulbs that it detects or otherwise replace a human, it can log and help dispatch one. The project was designed to collect data points and typically, with this kind of thing when reams of data are collected, photos end up being dumped into an unorganized grouping of folders and are difficult to view and make sense of. We felt it was important to provide structure to the information, and so we worked to feed these data points into an application that we would develop, called Autowalk Mission Evaluator (AME). It would allow an STM employee to efficiently and remotely review the data after Spot had completed its run.
The AME is not only a place to view the data in an organized way, but it provides a place to capture feedback (something that we felt was missing in the market). It also applies a layer of artificial intelligence to automatically analyze the anomalies. For example, trash as an object is actually a complex thing to identify with computer vision technology, as there are great variances between a pop can, a sticker, a crumpled up candy wrapper, etc.
This sort of object detection is reliant on a model that understands what things are (and what they aren’t). In order to add those items, we first have to give it a plethora of images to train it. So we first had to take many photos, annotate them, give them a label, and serve it back to the model for its initial training. With the feedback which would then be collected through the AME, a feedback loop would be used to improve the model’s performance and understanding of new anomalies. The AME applies a confidence score to each object so the person reviewing can get a sense of whether they should take a closer look.
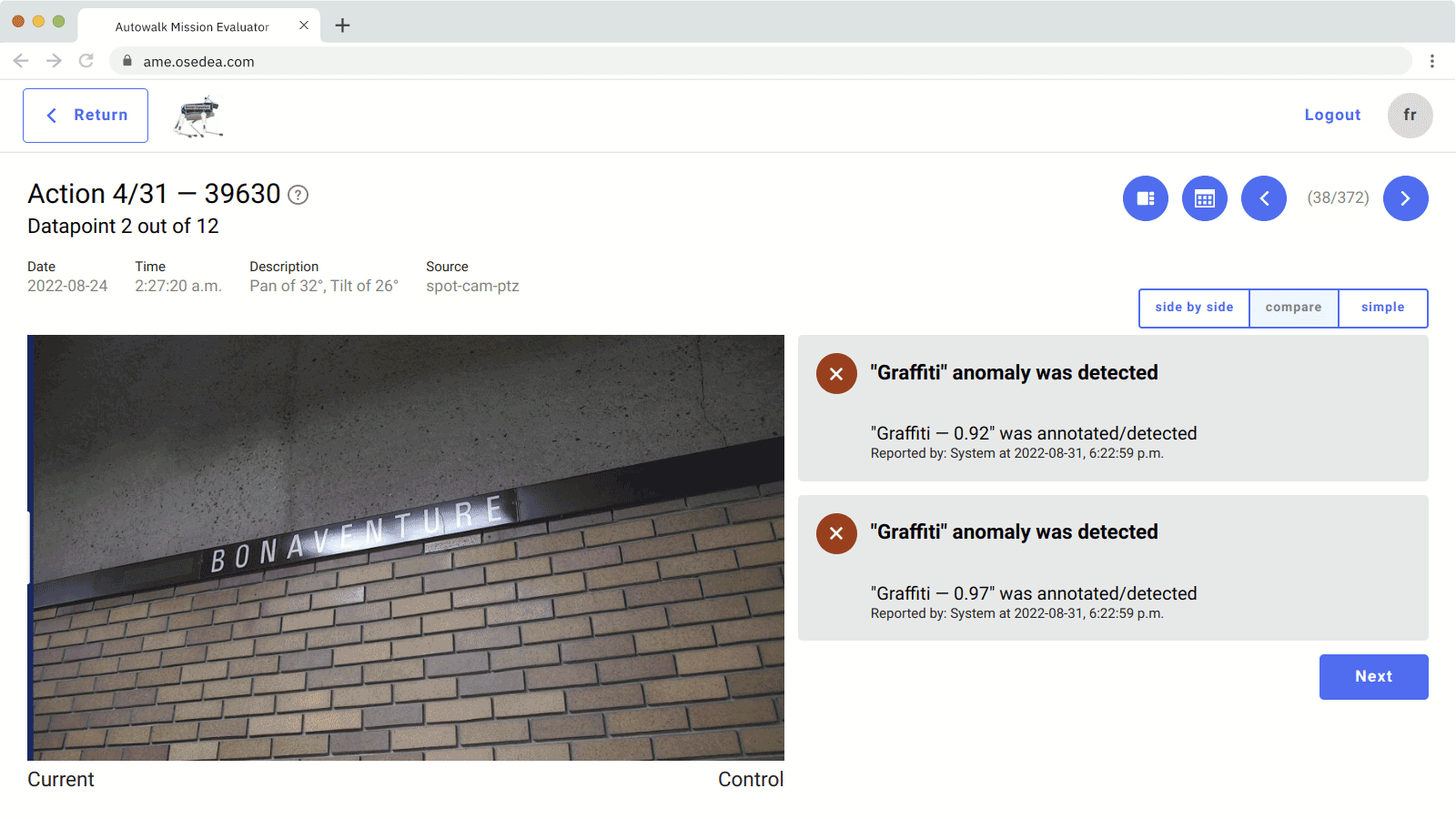

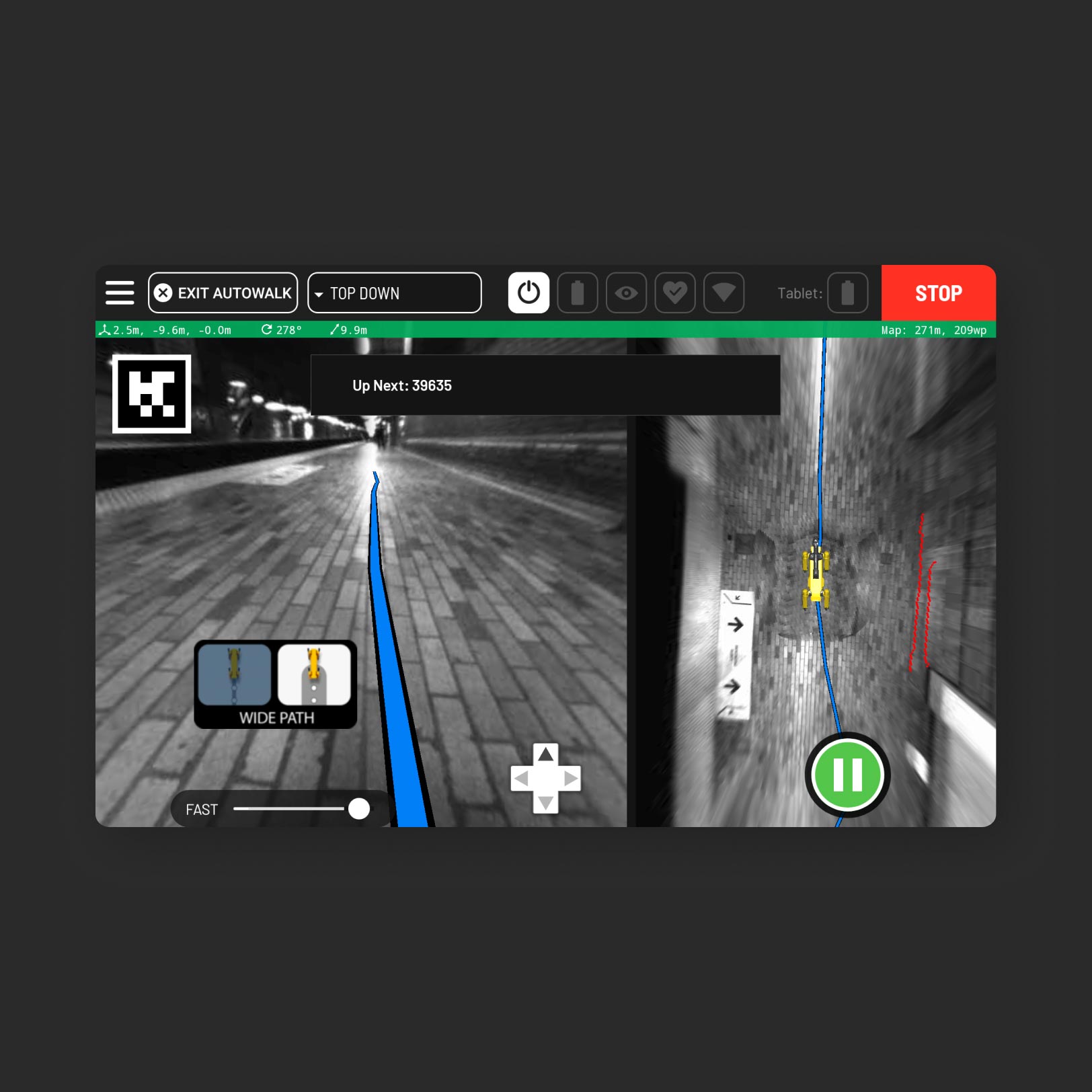


The end result
The purpose of this project, which took place between May and September, with Spot visiting the station about ten times over a period of two months to perform its duties, was for the STM to become familiar with the technology, demonstrate Spots capabilities, and explore the potential of what this autonomous robot is capable of. The results were very interesting and both we and the STM are pleased with how it went. This is especially true because we started with such a low volume of training data and, as mentioned above, trash, stickers and graffiti are all complex for computer vision to identify.
Ultimately, with Spot we were able to detect a lot of trash and anomalies on the platform. The robot achieved 86% coverage, compared to that of a human. And, for correct object detection in the AME, we achieved about 70% accuracy, with no human intervention. It's like having an apprentice who learns the trade, until becoming 100% autonomous and freeing the employee for these tasks, which is a great demonstration of the potential of autonomous automatic robotics. With the Spot platform deployed, additional functionalities can be added onto its runs without loss in quality or efficiency in order to add to the ROI it brings. For example, having a human take several photos every few feet, while not ideal, is possible. But having that human carry additional sensors to record air quality data at the same time, is likely a step too far.
The STM is an innovative and forward-thinking organization that is always looking to improve the experience of their clients. In their case, they’re not looking to replace employees, but to make their lives easier so they can focus on more important tasks.
What’s next
While the data from the pilot project had useful applications in the moment (e.g. it could be leveraged to dispatch a janitor to deal with trash, sticker and graffiti cleanup, or to dispatch a maintenance worker to swap out a lightbulb), its real value lies in its consistency and insights that can be extracted from historical data. The STM could, in theory, leverage sanitation data to identify trends (e.g. what day of the week accumulates the most trash, stickers and/or graffiti, and approximately how many lightbulbs burn out per month), which could in turn impact staffing decisions. And, because Spot can be equipped with other sensors, there is also potential for extensions. In place of a camera, for example, sensing equipment could theoretically be added to capture the air quality in the metro, or Spot can check the health of rails and tunnels for cracks and deterioration.
Even though our experimental project was primarily aimed at the integrity of the station for the customer experience, we believe that there are other sectors at the STM where Spot could help, such as for the fire department, construction and progress monitoring of these projects as well as for the security service. The possibilities are really endless!
Spot Robot cannot be leased out from Boston Dynamics to test out. But we have one! This means that companies like yours can do a pilot project with Spot, which is an incredible and low risk opportunity to experiment with high-end, state of the art technology for a pilot project that lasts a couple of days, weeks or months. The choice is yours. Get in touch today to chat about your vision.

Insights
We’re on a constant quest to broaden our horizons and spread wisdom. It’s all about pushing boundaries and elevating our game.